GIS
Fusing the Boundaries of Spatial & Non-Spatial Data Management (PART 1)
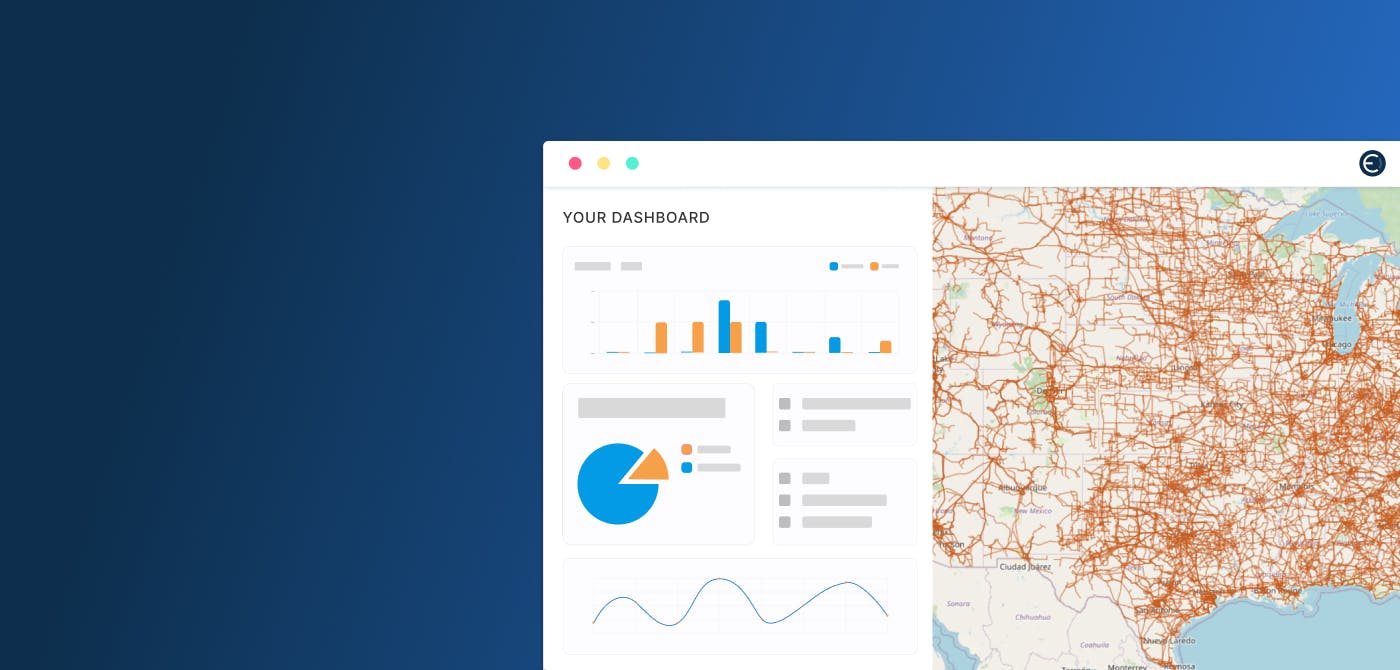
Liked what you read?
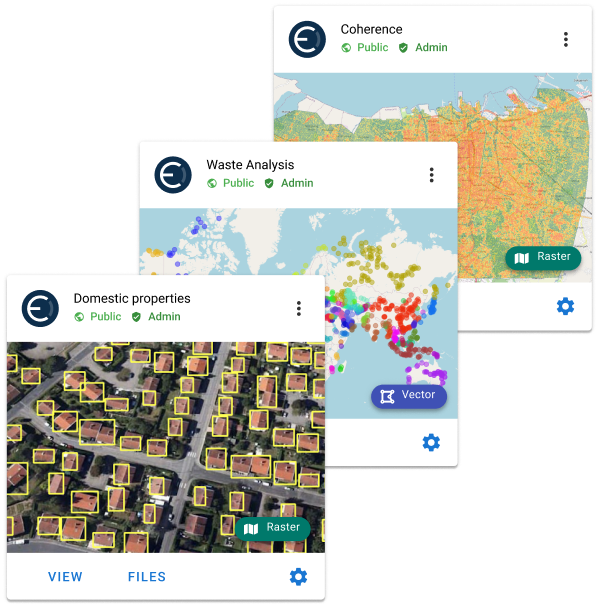
Subscribe to our monthly newsletter to receive the latest blogs, news and updates.
Contents
The Status Quo
There are countless solutions available for general (non-spatial) data management, ingestion, integration and analysis–Databricks, Snowflakes, BigQuery being some of the well known names that spring to mind. But there are no satisfactory solutions like these available for spatial data.
The current lot of commercially serviced spatial data infrastructures have a baseline fee ranging from hundreds of thousands up to millions of dollars. To top that off, they come with perpetual lock-ins and interoperability fees. It leaves the impression that they’re in the money making business, not the data democratization business!
The build-it-yourself option– using GeoServer, PostGIS or Protomap is also not ideal. With this option you pay in the form of development cost and inefficient workflows that involve tech teams being burdened with ad hoc patching and data wrangling that slowly eats away at your bottom line.
The end result of both these routes? Spatial data is woefully siloed. Even neglected in strategic decision making processes in order to avoid dealing with the data management issues stemming out of it.
Is it worth merging the boundaries between spatial & non-spatial data management? Let’s validate this by looking at a few future trends in the ecosystem!
Future Trends in the Ecosystem
- Growth of Remotely Sensed Data – There is a growing influx of geospatial data through drones, satellite constellations and IoT devices. Not only is the quantity of data increasing, the quality and variety is also becoming more sophisticated (eg. Radar, Lidar, Hyperspectral imagery). This wealth of information needs the right infrastructure for it to be leveraged effectively.
- AI and Machine Learning – AI and machine learning technologies are getting better at churning out predictive models and real time analytics that enable dynamic, location-informed decision making.
- Growing Industry Adoption – Location data is pivotal to pretty much every major industry on the planet (retail, insurance, agriculture). This fact is being acknowledged by an increasing number of business leaders. Organizations are looking for ways to incorporate spatial data into their strategic decision making processes.
All of these trends point to one conclusion - Spatial data is here to stay and it is vital that we learn to harness its true potential by creating a conducive infrastructure for it.
Despite a unanimous conclusion, there seem to be several blockages that prevent the seamless integration of spatial and non-spatial datasets.
Challenges of Integrating Spatial & Non-Spatial Datasets
Manual Integration → Spatial data inputs entering analytical workflows look different each time. This creates an integration challenge that requires a lot of manual intervention.
Capital Investment → As briefly mentioned at the top of the article, both the (build and buy) options available come with a heavy price tag. And despite the price tags, they are anything but ideal.
Siloed Data Ownership → In many organizations, spatial data and non-spatial data are managed by different teams, leading to fragmented workflows and ineffective data integration.
Expertise Gap → There is a clear lack of data professionals who are skilled at working with both spatial & non-spatial datasets. This lack of in-house geospatial talent increases the project cost.
Data Governance → Fusing spatial and non-spatial datasets implies aligned master- and metadata governance policies, FAIR Principles, and proper access controls. Good luck with that!
A Seamless Integration of Spatial and Non-Spatial Data
So what does a conducive & seamless environment – that bypasses most of the above challenges – look like? And how does one go about creating it?
In essence, any type of spatial data - of any size - must be queryable and computable with the same/similar tools and methods as non-spatial datasets. Easier said than done. You can’t use Databricks to query raster data, can you? You need a different set of tools to get spatial data out of its silo.
Creating web services is a great starting point to break free spatial data. It allows you to interact (i.e. search, render and visualize) with spatial data in a low threshold way. Quite similar to the way in which you work with non-spatial data as a web service.
That’s one of the main features of Ellipsis Drive - a fully interoperable, cloud based, spatial data management solution. Ellipsis Drive automatically turns any spatial data that you have under management into a high performance and interoperable web service. It removes the need for all the additional ad hoc data wrangling work that is normally required from tech teams and allows you to query web services quite similar to the workflow on Databricks for non-spatial datasets.
With the right infrastructure you can slash your spatial data related woes in half. If you want to go all the way, get in touch with our technical team for a detailed discussion of your use case.
Take the Ellipsis Drive tour
in less than 2 minutes’
- A step-by-step guide on how to activate your geospatial data.
- Become familiar with our user-friendly interface & design
- View your data integration options
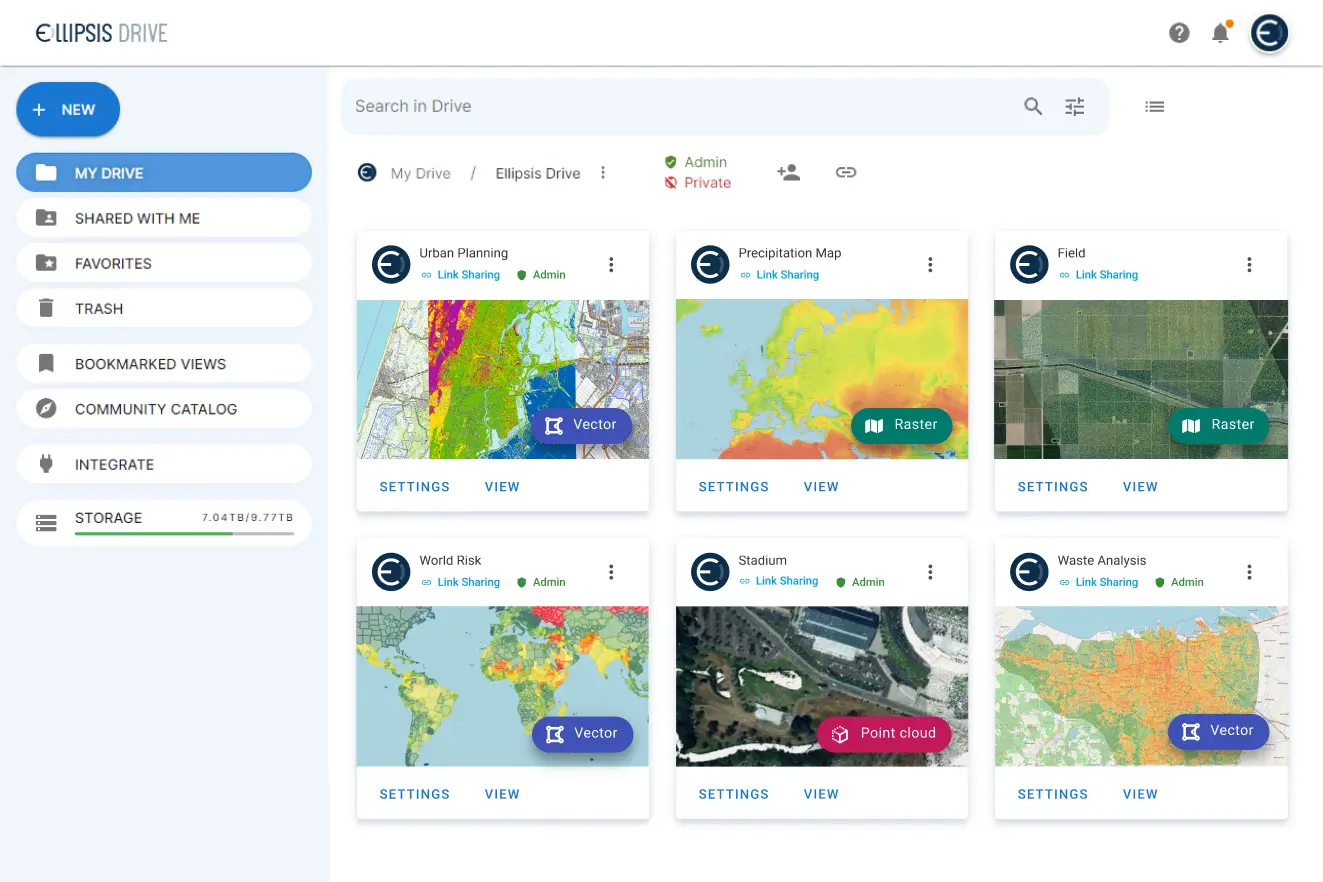
Related Articles
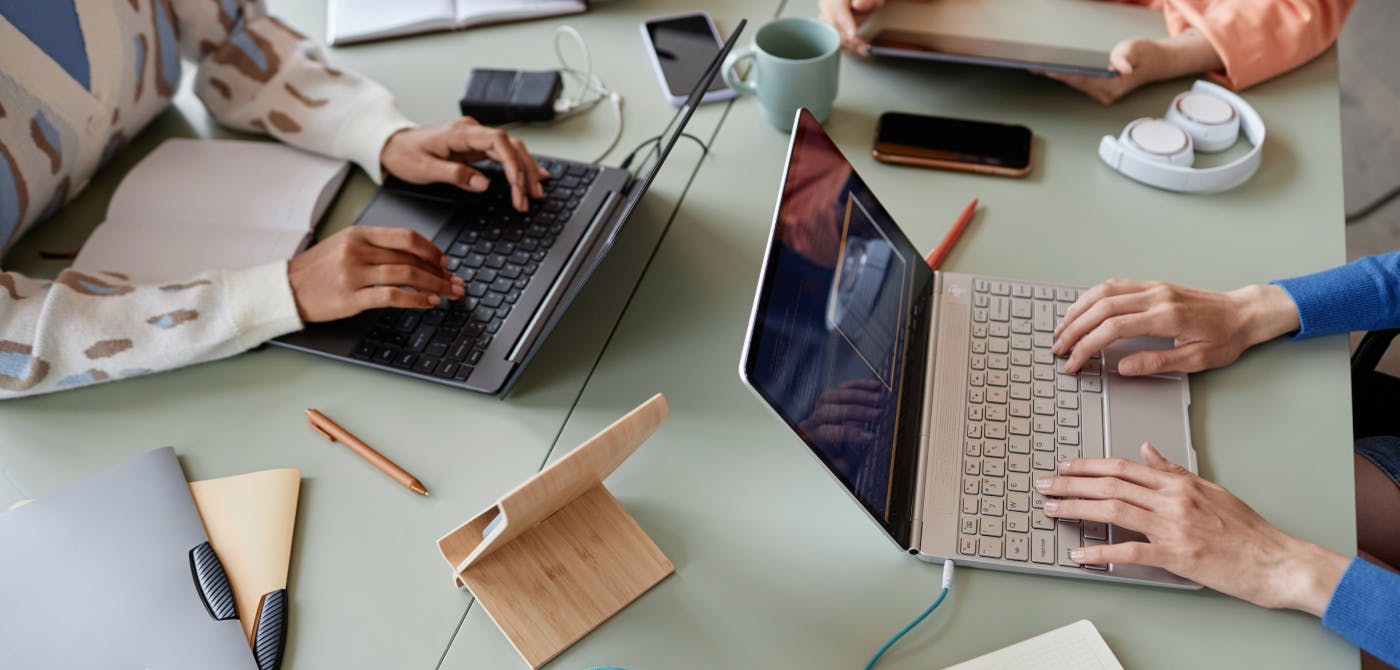
Unlocking the Potential of Data: Comparing Tabular and Non-Tabular Protocols
The world of data is a complex landscape and each year, the complexity of that landscape grows exponentially. A combination of new data capturing technology, data processing technology and demands fo
6 min read
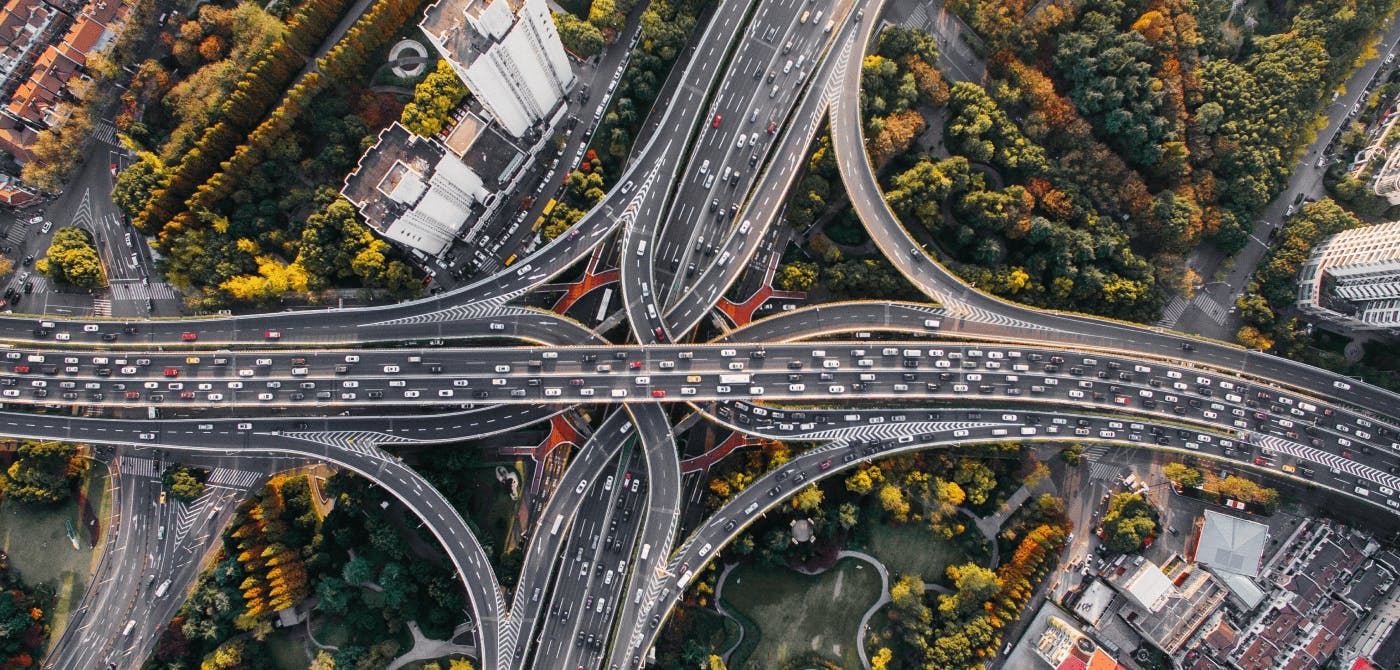
Understanding the importance of GIS in Urban Planning
Cities are robust places filled with life, but before it becomes a cosmopolitan paradise, plenty of ever-evolving complexities are happening behind the scenes to bridge the gaps and create an area spa
3 min read
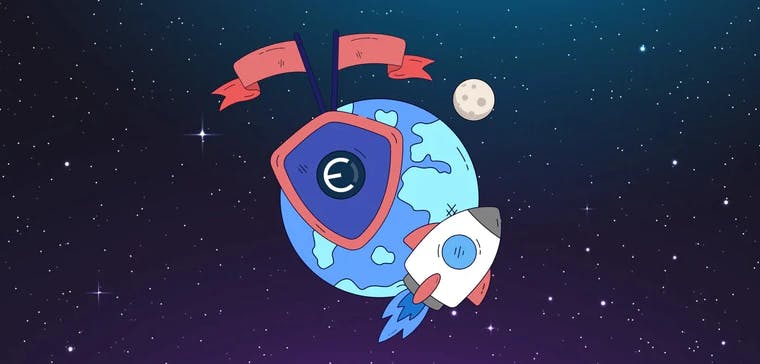
Ethics in GIS - An Overview
As the future of digital systems looks bright, Geographic Information Systems (GIS) is becoming more popular in various industries to help companies map all types of data. The main goal of GIS is to c
3 min read