Use Case
Deep Learning for Deforestation Alerts
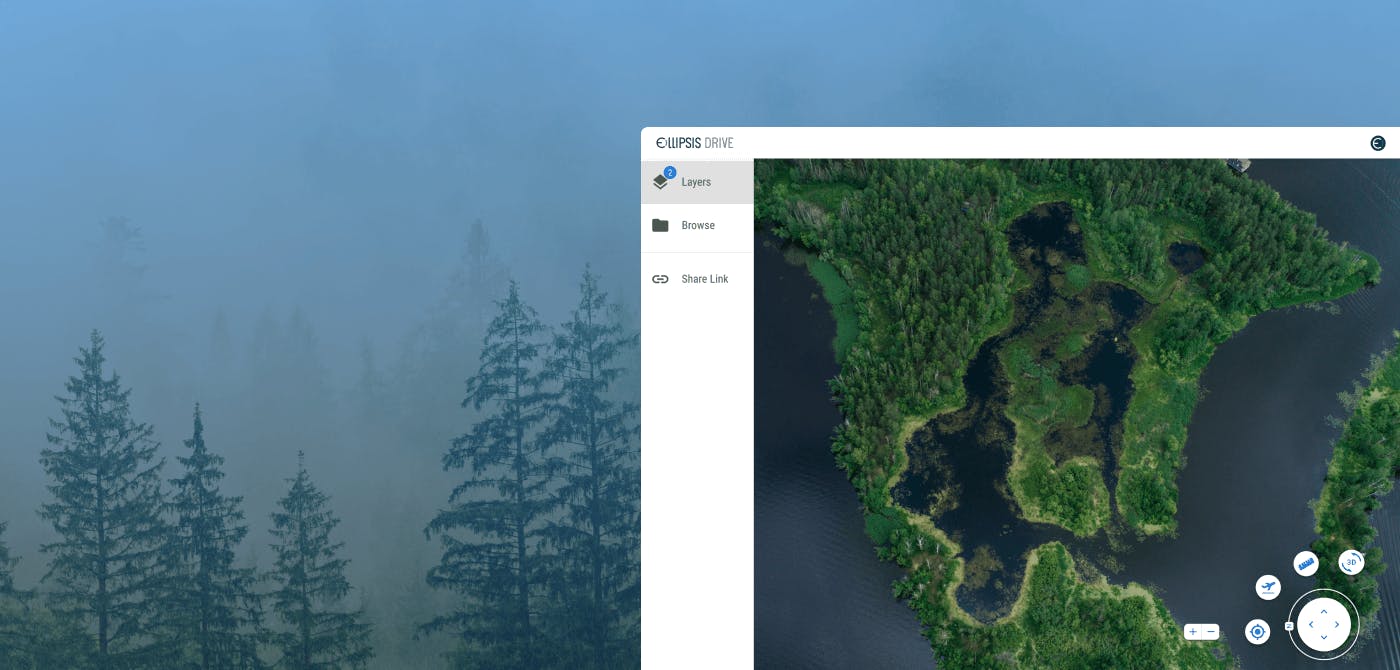
Liked what you read?
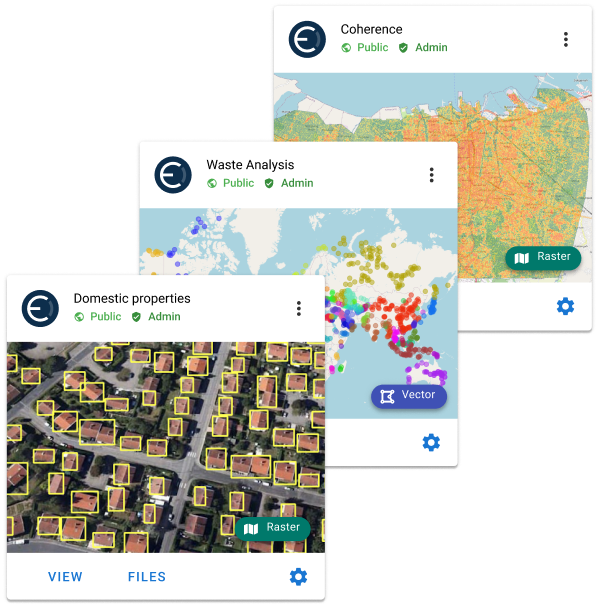
Subscribe to our monthly newsletter to receive the latest blogs, news and updates.
Contents
A collaborative initiative to identify drivers of deforestation
Global Forest Watch (GFW) offers free, near-real-time data, technology and tools for monitoring the world’s forests, enabling better protection against illegal deforestation and unsustainable practices. One GFW offer is the satellite-driven near-real-time deforestation alerts, produced by the University of Maryland and Wageningen University & Research. Such alerts detect tropical forest disturbances on a weekly basis, giving forest monitors an idea of where deforestation may have occurred. The alerts detect the loss or removal of trees.
However, they currently do not indicate the driver behind the loss. GFW partnered with Science [&] Technology AS (S&T) and Ellipsis Drive to develop a prototype method to classify deforestation alerts by their likely drivers in near-real time. S&T excels in technology solutions and applied their expertise in advanced machine learning (deep learning) techniques.
The collaboration was successful, with iterative model development between the domain experts at GFW and the Earth Observation-Machine Learning S&T team, who designed the pipeline, created and refined the models. Ellipsis Drive provided a platform through which both parties could visualize spatial data and comment on drivers in specific locations, facilitating communication and model improvement.
Training data and model development
During this pilot project, S&T classified land cover/land use using Sentinel-2 imagery in West Kalimantan (Indonesia) from a specified time range when deforestation alerts were detected. They created a deep learning model for semantic segmentation in order to identify patterns of selective logging, mining, small- and large-scale agriculture, and fire.
Since fire is often used for the purpose of clearing forest to convert land for another use such as agriculture, S&T developed a double-headed model with the capability to predict the driver of the alerts and determine whether fire was involved in the forest clearing.
GFW used the Collect Earth Online platform to streamline the training data collection and referenced high-resolution imagery to label the deforestation alerts with their corresponding driver and collect additional polygons. Once the training data was uploaded into Ellipsis Drive, S&T used the comment feature to leave messages associated with certain locations. The partners explored and discussed these locations using the “zoom-to” feature in Ellipsis Drive.
Throughout the model iterations, S&T consistently generated reports on the model performances, noting any augmentations they made, such as geometric transformations, color changes, blurring and sharpening. One of the challenges of using optical remotely sensed data in this geographic region is the persistent cover of clouds, often obscuring the deforestation patches and the context around them. S&T experimented with various cloud masking techniques and incorporated artificial clouds and haze into their methods to account for the presence of clouds.
The performance of the land cover prediction and the driver classification were assessed for each model iteration, thereby tracking model improvement over the course of the project.
Towards prioritizing forest loss alerts for action
This work resulted in a well performing model, which was able to predict drivers including selective logging and small-scale agriculture. Further work is required to test this method beyond the study area, and to improve its performance. Potential next steps include generating more training data to address confusion between classes such as new roads and selective logging.
Identifying drivers of forest loss in near-real-time could be vital for forest monitors, allowing alerts to be prioritized and resources focused for monitoring and response.
Ellipsis Drive is glad to be part of such initiatives and is happy to contribute to the conservation of the environment.
Take the Ellipsis Drive tour
in less than 2 minutes’
- A step-by-step guide on how to activate your geospatial data.
- Become familiar with our user-friendly interface & design
- View your data integration options
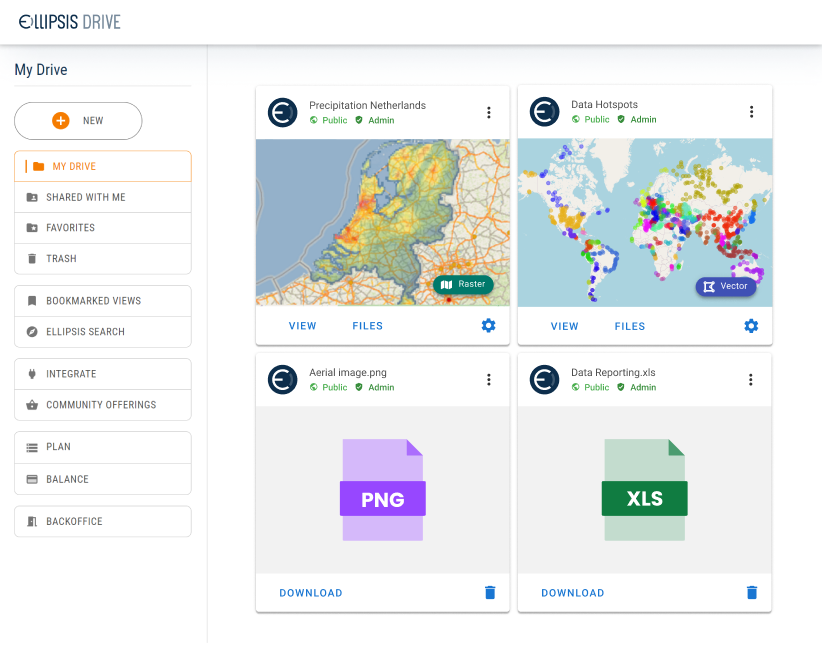
Related Articles
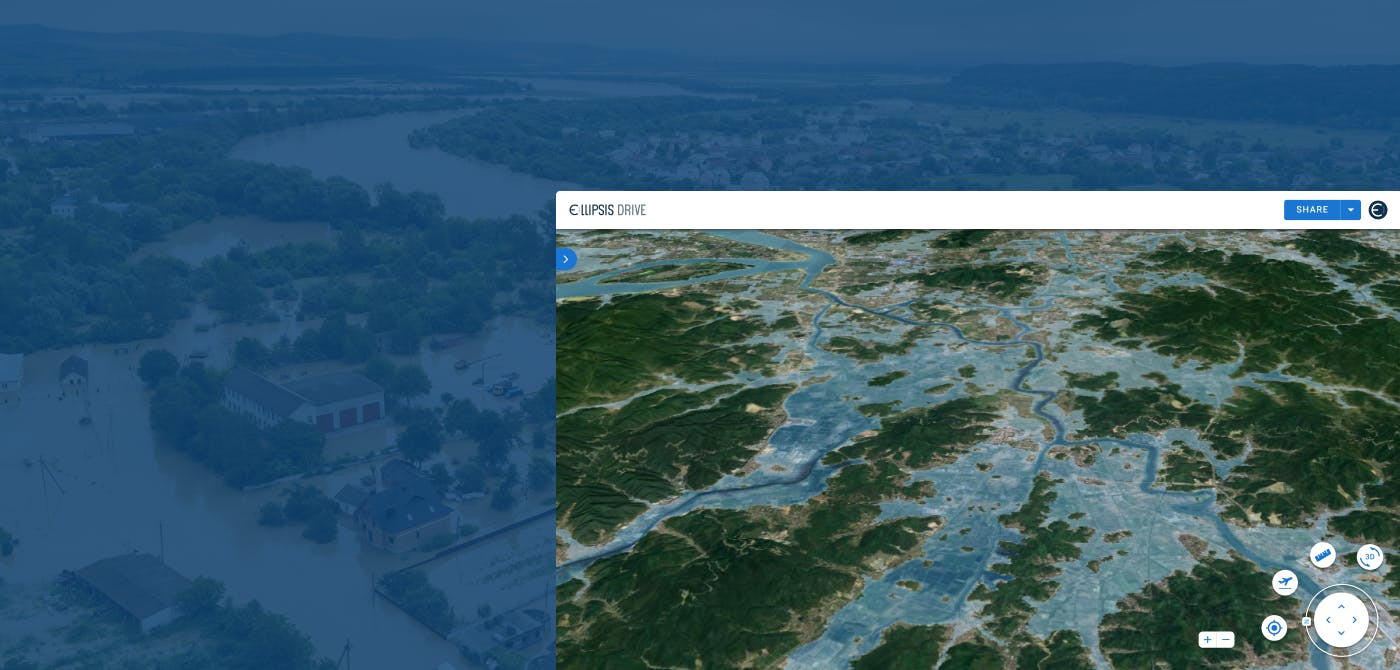
JBA Consulting | The Pursuit of Resilience Against Climate Change
In the late 2000s, the Environment Agency of England and Wales assigned an environmental engineering consultancy with an apparently impossible task - to create a flood map for the entire country in
5 min read
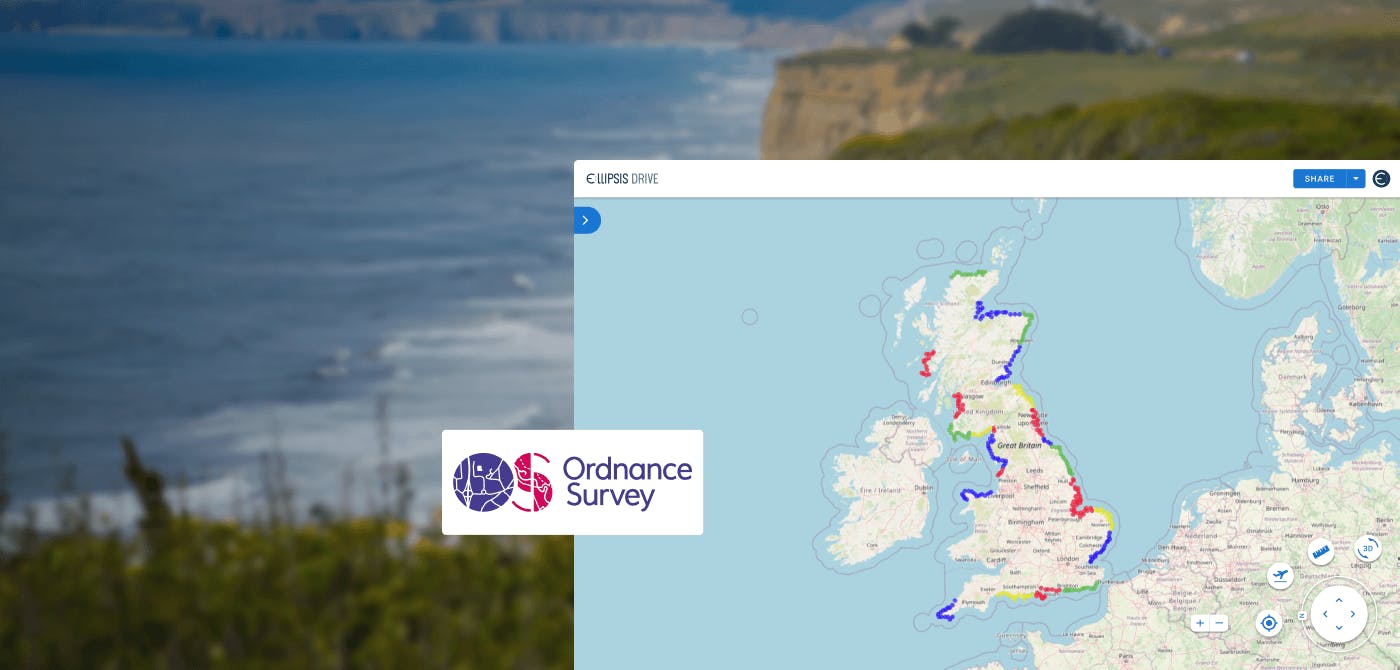
Ordnance Survey's Geovation Connects Industry Challenges to Tech Start-Ups
Geovation is a UK based organization that plays a crucial role in promoting and accelerating the growth of location, property and geospatial industry startups. It is an Ordnance Survey (the national
4 min read
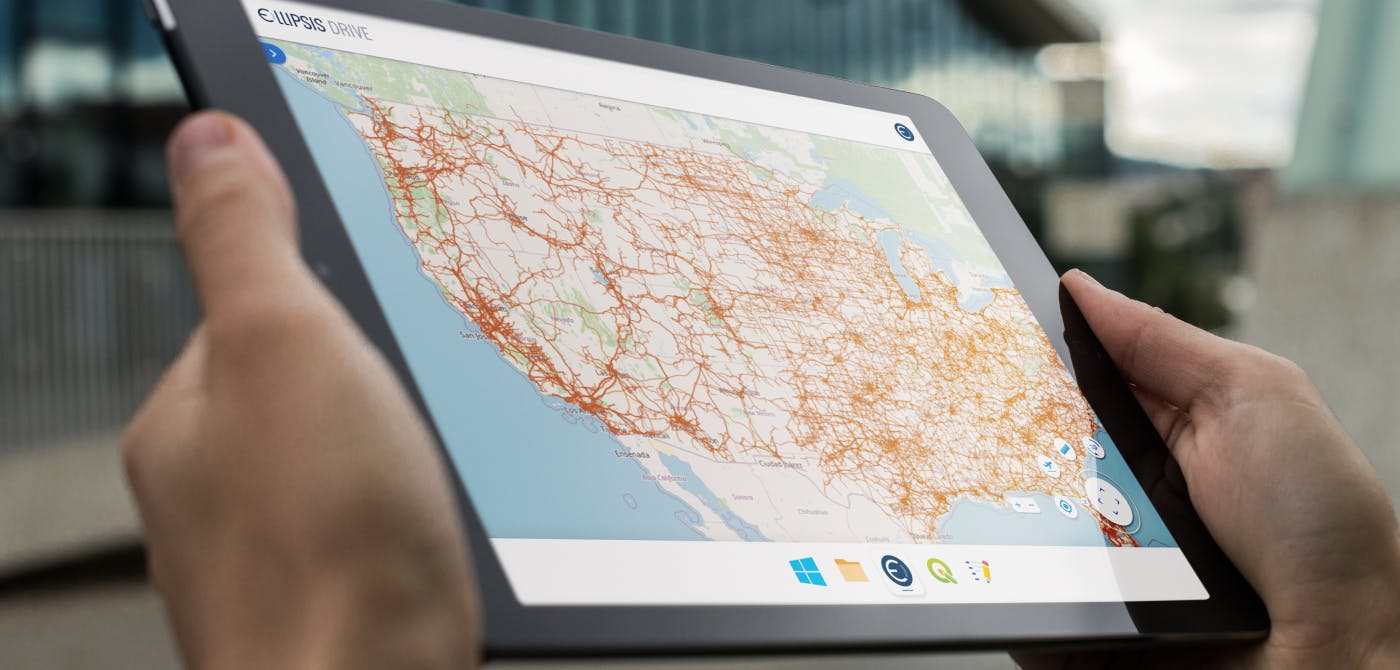
Getting the Most Out of Your Spatial Data Management Solution Ft. Ellipsis Drive
Let’s paint 2 distinct (yet very realistic) scenarios that occur prior to the sale of spatial data related products. The first scenario involves a sales team incessantly badgering their solution eng
5 min read